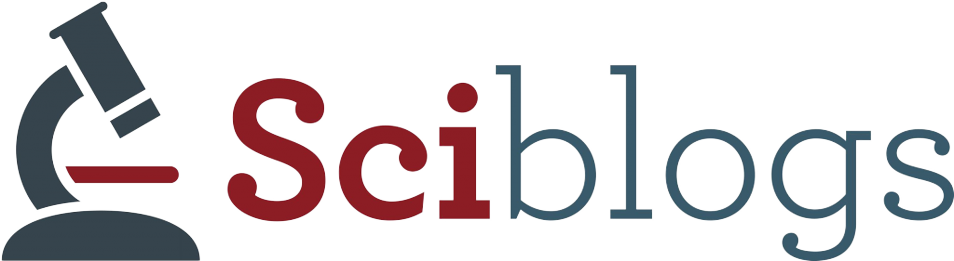
After 13 years of hosting Aotearoa New Zealand’s best science blogs, Sciblogs is now closed. A full archive of its content is available here:
Read Sciblogs Editor Dr Sarah-Jane O’Connor’s post bidding farewell to Sciblogs.
Read Sciblogs co-founder Peter Griffin’s post on the history of Sciblogs.
Help – my link to a Sciblogs post has broken!
To redirect any links, simply add the National Library archive address in front of your link,
For example, to get to the main Sciblogs page:
Copy:
https://ndhadeliver.natlib.govt.nz/webarchive/20220622093355/
Paste:
https://sciblogs.co.nz/ to the end of the URL above
Result:
https://ndhadeliver.natlib.govt.nz/webarchive/20220622093355/https://sciblogs.co.nz/
For all inquiries, please contact smc@sciencemediacentre.co.nz